What Are the Techniques to Overcome the AI Fraud Detection
Identifying fraud is a significant obstacle to innovation when it comes to hiring artificial intelligence consulting companies. This is since only a small portion of financial transactions are fraudulent; locating them comparable to searching for a needle in a stack of needles. Fraudulent transactions are complicated to pinpoint using rule-based structures since it is almost impossible to define any suspicious transaction without AI consulting services. We try to detect deviations from expected behavior by knowing what “natural” for the AI managed services is.
Additionally, ML models are adaptive, and so organized crime tactics often change. An AI model is poised to respond to dynamic anomalies which represent fraudulent patterns. Traditionally, auditors and financial institutions also favored machine learning for fraud detection because of these considerations.
The last year has seen a marked increase in the pace of this epidemic, and it culminated with Amazon making fraud detection tools widely accessible to artificial intelligence consulting companies. The increase in fraud detection technology is in no small part due to the Covid-19 outbreak.
A growing trend in fraud that demonstrates reduction incapacity
Between 2020 and 2024, it is estimated that losses from digital money fraud will increase by 130 percent. By 2024, it is estimated that fraudulent transactions will total $10 billion. This trend accelerated significantly during the pandemic’s first period, with a 6% increase in digital fraud toward businesses between March and May. Fraudsters have attempted to capitalize on the abrupt transformation companies and workers experienced earlier this year and the resulting artificial intelligence consulting companies inefficient in handling communication disruptions.
Simultaneously, many of the fraud-monitoring teams were forced to transition to remote working later this year quickly, and many companies put on several others furlough. This suggests that while there was an increase in fraud – which would put teams to the test even under normal business circumstances – anti-fraud teams found themselves dealing heavily with artificial intelligence consulting companies that were short-staffed and operating in an unfamiliar climate.
This made the pandemic an ideal opportunity for many companies to accelerate the deployment of artificial intelligence-based fraud detection platforms. Although increased adoption of AI models for fraud detection systems was inevitable, the pandemic has intensified this trend by providing a short-term incentive for businesses to automate and redefining industries with AI.
Challenges facing AI for fraud detection
Challenges that arise for teams in AI in the fight against fraud are unfamiliar, including deploying and scaling it in places where fraud is prevalent and detecting it as it. Adding AI to teams often generates regulatory, legal, and ethical issues.
Another difficult challenge is explicability. Teams should comment on the various signs of a fraudulent transaction regarding fraud detection and proof. A poorly implemented AI may have the potential to expose the explanation. Since neural networks comprise several layers of technical representations, they can be more complicated and challenging to comprehend without leveraging artificial intelligence consulting companies.
Any discrepancy creates the possibility for an AI to begin detecting false-positive events, with automated systems unable to investigate the AI’s reasoning. This might potentially harm ordinary consumers trying to make an ethical investment, driving them away from the project.
Another issue is that without proper creation and function, an AI can make predictions biased in a negative direction. If it goes untreated, these undesirable prejudices may develop into overt discrimination based on ethnicity, sex, or other covered entity. This is because an AI’s decision-making process is primarily determined by the data it is “educated” on. If the training set is unrepresentative or skewed in any way by artificial intelligence consulting companies, the AI will inherit these prejudices and make judgments accordingly.
Bringing humans into the loop
The bias problems are likely to be challenging and pressing for many financial service providers, even more so given that many would have had to rapidly scale up their AI fraud prevention platforms in response to the crisis. Fortunately, there are resources and best practices available to assist teams in ensuring their models stay explainable and free of bias, such as automated analysis strategies and libraries that help in explaining and codifying precisely what occurs within the AI’s “black box.”
Another practice that is well-suited to addressing these issues is the “human-in-the-loop” testing and deployment model. This requires humans to carefully curate the data used to train the AI, actively participate in tuning the AI model to improve its predictions, and regularly monitor its output. When introduced, a human-in-the-loop structure advocates that a responsible artificial intelligence consulting company is never more than a few steps removed from an AI’s decision-making process, allowing for monitoring and ensuring that the AI’s decisions are explicable and ethical. Additionally, human-in-the-loop means that everyone is responsible for the decisions taken by AI platforms for fraud detection.
The recession has made it an ideal time for deploying AI in business intelligence for identifying fraud. A revolution, not immediate but long-term, has been accelerated by the imperative of economics. Adopting good practices, such as humans-in-the-the-the-loop, companies like financial service firms will show that their AI systems have limited teething issues and significant long-term benefits.
Share This Post
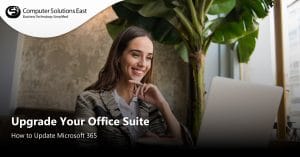
Upgrade Office Suite: How to Update Microsoft 365
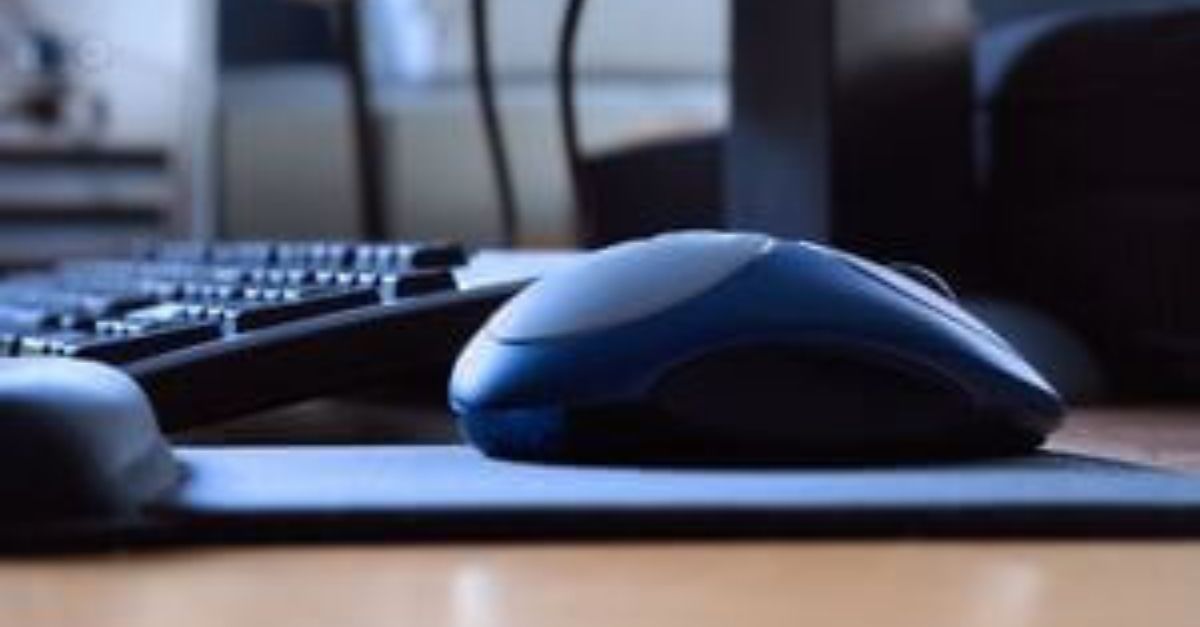