ML and Cyberattacks: The Recent Trends Showcasing Why Cybersecurity Is Crucial
In crucial areas such as finance, health care, and defense, Machine Learning (ML) is witnessing an incredible transformation, affecting almost every part of our lives. Many firms who wish to capitalize on ML advances have not tested the protection of their ML programmers. Microsoft also launches the Adversarial ML Threat Matrix with an industry-focused open platform and contributions from multiple enterprises. It enables security researchers to recognize, respond to, and remediate attacks against ML systems to ensure robust cybersecurity services.
Even if machine learning technologies are commonly deployed in critical fields like banking, healthcare, and security, companies’ protection has not been taken care of, which Microsoft is now aiming for. In recent years, Microsoft has seen a spike in attacks on commercial ML devices. In the Gartner report, 30 percent of all AI cyberattacks are expected to leverage training data poisoning, AI model stealing, or opponent-powered systems.
The survey suggested considerable cognitive dissonance, particularly among security analysts who typically think the danger to ML systems is a futuristic issue. This is how cyberattacks on ML networks are modified, which creates a concern. In 2020, for example, enterprises are witnessing their first Common Vulnerabilities Exposure (CVE) with a lot of malware-based attacks.
The first vulnerability was released by SEI/CERT, pointing out the number of arbitrary misclassification attacks by existing ML systems that assault security, credibility, and availability. Since 2004, the researchers are raining the alarm that repeatedly demonstrated that ML networks could be breached if they are not secured.
Microsoft has collaborated with MITRE to produce the Adversarial ML Threat Matrix because the first move enables defense teams to protect themselves against ML systems threats. This is possible by coordinating tactics that hostile opponents use to subvert ML systems. We expect the security group to use tabulated tactics and techniques to improve their surveillance methods around the enterprise’s vital ML structures.
The primary audience is security analysts
Securing the ML systems needs top-level cybersecurity services in the USA. The aim of the Adversarial ML Threat Matrix is for security analysts to place attacks on ML systems to counter these new threats. This matrix is widely accepted in security analysts’ culture, where enterprises can leverage their services with a different framework to tackle ML systems threats. Adversarial ML Threat Matrix often varies significantly from conventional attacks on corporate networks since ML systems’ attacks are fundamentally different.
Grounded in real attacks on ML Systems
Computer Solutions East works closely with Microsoft and pivots the team to place secured ML architecture with various flaws and adverse actions. This is to tackle ML virtually systems output with Security researchers focusing on reducing practical risks to ML programs.
There is a need for cybersecurity services in the USA that provides a learning system with a comprehensive experience. A team can also orchestrate real attack simulations to help navigate the prospective attacker’s end goal, contributing to more insidious model evasion.
It is also possible that attackers use a mix of traditional tactics’ including phishing and lateral motion, alongside adverse ML techniques when targeting an ML device.
Microsoft contributes to stable ML systems development and implementation by integrating the tools and resources necessary for securing the ML systems. Having a reliable team of engineers at CSE helps advise to build safe, secure, and reliable ML solutions that sustain customer interest.
Extending Cybersecurity Services to ML
The Azure Trustworthy Machine Learning team at CSE reviews the security stance of vital ML systems regularly. This is possible as we partner with Microsoft at the product level to offer front-line defenses using Microsoft Security Response Center (MSRC). This way, businesses can thoroughly secure and track ML systems from active attacks. Multiple individuals regularly share the lessons of these practices with the community:
Microsoft provided a taxonomy describing a variety of ML failure modes to engineers and policymakers as following:
- Publishing recommendations for ML systems specifically for threat modeling to be used by the developers.
- Enabling businesses to launch our bug bar to triage attacks on ML systems for security incident respondents systematically.
- Microsoft has opened a $300KAI Safety RFP for academic researchers and has partnered with many universities to push this into practice with a good feasibility run.
- Microsoft organized a practical evasion learning competition for business practitioners and safety professionals to build muscle in defense and attack ML systems.
The ML devices do need an extra bit of protection, driving safety analysts, and a more comprehensive safety community. Having the matrix and the case studies are intended to reinforce a plan for ML security and detection. The ML-systems go through simulated attacks at various levels to become possible for the enterprise real-time attacked. Similar exercises can be performed in their organizations carefully and validate the monitoring techniques.
Share This Post
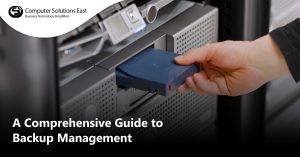
A Comprehensive Guide to Backup Management
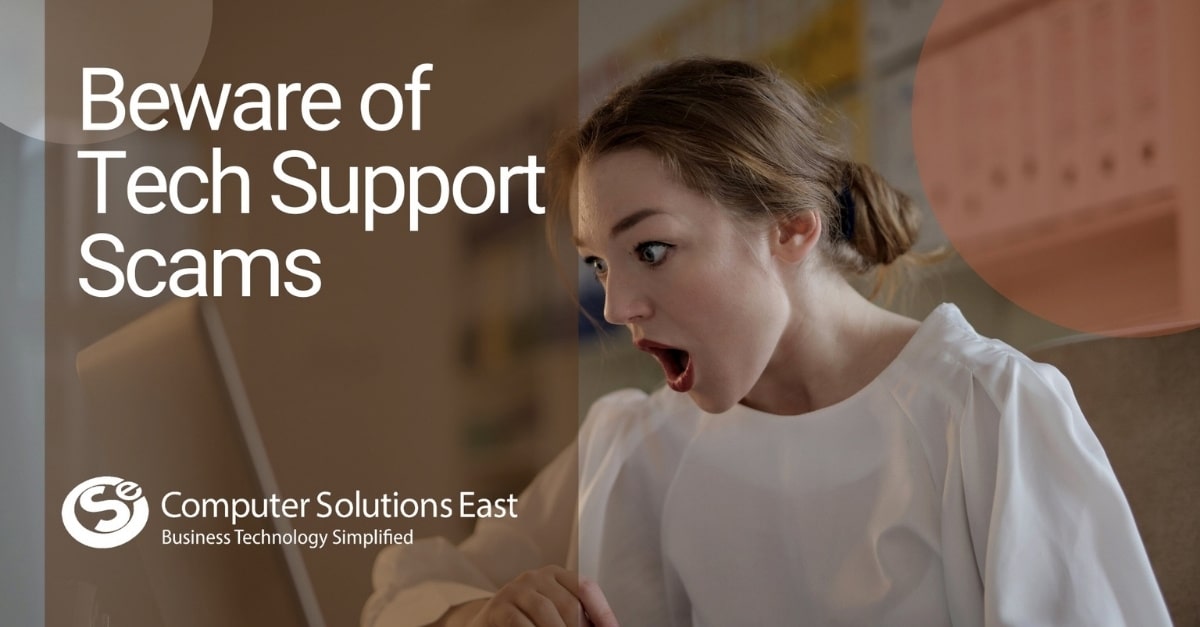